Dr Srijit Seal leads study to define AI-powered toxicology guidelines
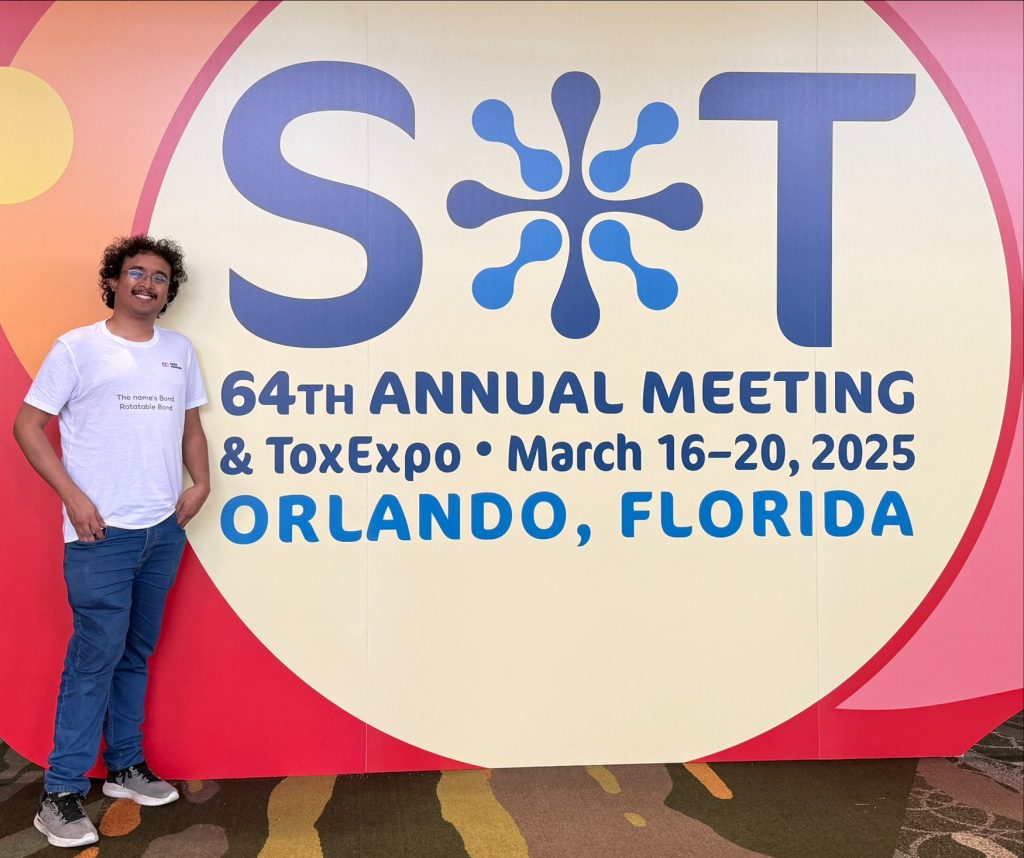
Dr Srijit Seal, who completed his PhD at Clare Hall, has recently led a landmark collaboration between over 30 scientists from pharmaceutical companies, government agencies, academic institutions, and AI innovators to outline the essential pillars for using machine learning (ML) to predict chemical toxicity. Published in ACS Chemical Research in Toxicology, the review, “Machine Learning for Toxicity Prediction Using Chemical Structures: Pillars for Success in the Real World,” comes in response to the US Food and Drug Administration’s plan to phase out animal testing, and the growing global momentum toward non-animal testing strategies.
The review proposes a five-pillar framework for reliable, actionable toxicity modeling, and draws from the expertise of scientists from companies including GSK (GlaxoSmithKline), Novartis, Eli Lilly & Company, Merck Inc., AstraZeneca, Bayer Crop Science, Pfizer, Recursion, Novo Nordisk, Sanofi, Relay Therapeutics and others, as well as government experts from the National Institute of Environmental Health Sciences (NIH) and thought leaders from academia, including Cambridge and the Broad Institute of MIT and Harvard.
“This paper is a practical guide to actually using AI in decision-making—how to choose the right data, the right models, validate them properly, and apply them to real-world scenarios like compound deselection or risk assessment,” Dr Seal said.
According to Dr Seal, the article introduces a structured framework that moves the field from AI enthusiasm to applied rigor:
1) Dataset Selection – data relevant to the toxicity of interest, with sufficient biological context and quality
2) Structural Representations – that preserve chemical and biological signals
3) Model Algorithm – that suits the complexity and information richness of the data
4) Model Validation – retrospective and prospective validation to assess the applicability domain
5) Translation to Decision-Making – to integrate models into real-world workflows
As the US FDA and global regulators look to phase out animal testing in favor of human-relevant, scalable models, this paper marks a decisive step toward that future. Read the full publication here.